TensorFlow 2.0 is out! Get hands-on practice at TF World, Oct 28-31.
TensorFlow Ads
Since the TF2.0 API reference lists have already been made publicly available, TF2.0 is still in RC.2 version. It is expected that the final release will be made available in the next few days (or weeks).
What’s new in TF2.0:
The obvious different – The version. In Colab, you can force using 2.0 by:
try:
# %tensorflow_version only exists in Colab.
%tensorflow_version 2.x
except Exception:
pass
#TensorFlow 2.x selected.
The latest version of 1.X and 2.X by the time of this article is shown below.
TF1.X
import tensorflow as tf
print(tf.__version__)
#1.15.0-rc3
TF2.X
import tensorflow as tf
print(tf.__version__)
#2.0.0-rc2
- Eager execution default – No more tf.Sesson()
Not long ago, I wrote a short tutorial for Graph and Session. Now, it has lost half of its value. TensorFlow 2.0 runs with eager execution (no more tf.session) by default for ease of use and smooth debugging. Consequently, sessions instantiating and running computation graphs will no longer be necessary. This simplifies many API calls and removes some boilerplate code from the codebase.
TF1:
with tf.Session() as sess:
outputs = sess.run(f(placeholder),
feed_dict={placeholder: input})
TF2:
outputs = f(input)
#No placeholder too
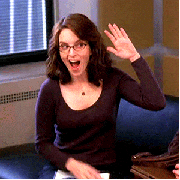
- tf.function decorator
The tf.function function decorator transparently translates your Python programs into TensorFlow graphs. This process retains all the advantages of 1.x TensorFlow graph-based execution and also you get the benefits of faster execution, running on GPU or TPU, or exporting to SavedModel.
This is the significant change and paradigm shift from v1.X to v2.0.
#TF1.X
def simple_nn_layer(x, y):
return tf.nn.relu(tf.matmul(x, y))
x = tf.random.uniform((3, 3))
y = tf.random.uniform((3, 3))
simple_nn_layer(x, y)
#output:
# tf.Tensor 'Relu:0' shape=(3, 3) dtype=float32>
#TF2.X
@tf.function
def simple_nn_layer(x, y):
return tf.nn.relu(tf.matmul(x, y))
x = tf.random.uniform((3, 3))
y = tf.random.uniform((3, 3))
simple_nn_layer(x, y)
#output:
# tf.Tensor: id=23, shape=(3, 3), dtype=float32, numpy=
array([[0.40271118, 1.0422703 , 1.0767251 ],
[0.8186165 , 1.4228333 , 1.5362573 ],
[0.6336632 , 1.1835647 , 1.1426603 ]], dtype=float32)>
Bye tf – Hi tf.keras
Keras has received much praise for its simple and intuitive API for defining network architectures and training them. It integrates tightly with the rest of TensorFlow so you can access TensorFlow’s features whenever you want.
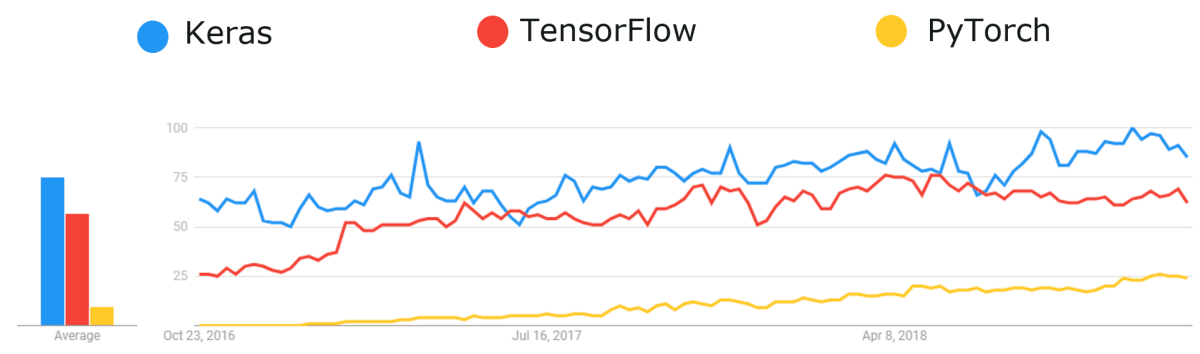
Importantly, Keras provides several model-building APIs (Sequential, Functional, and Subclassing), so you can choose the right level of abstraction for your project. With the increasing popularity of Keras, it is not difficult to understand that.
- tf.data
Training data is read using input pipelines which are created using tf.data. This will be the preferred way of declaring input pipelines. Pipelines using tf.placeholders and feed dicts for sessions will no longer benefit from performance improvements in subsequent tf2.0 versions.
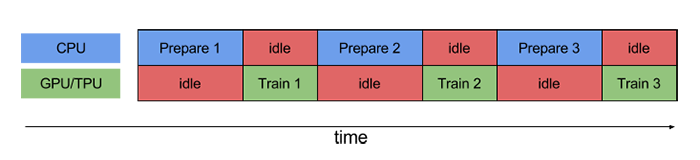
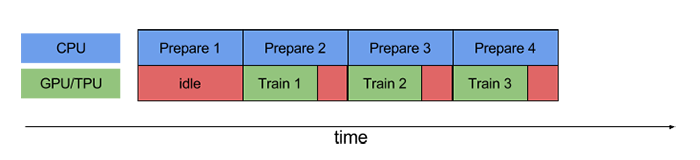
- R.I.P tf.contrib
Most of the modules in tf.contrib will depreciate in tf2.0 and will be either moved into core TensorFlow or removed altogether.
Migrate to TF2.X
It will still be possible to run tf1.X code in tf2 without any modifications. However, to take advantage of many of the improvements made in TensorFlow 2.0, you must convert it to TF2. Fortunately, the TF team does provide a conversion script that automatically converts the old tf1.XX calls to tf2 calls, if possible.
tf_upgrade_v2 \
--intree my_project/ \
--outtree my_project_v2/ \
--reportfile report.txt
Example of converted code from 1.X to 2.X:
# Code in TF1.X (before conversion)
in_a = tf.placeholder(dtype=tf.float32, shape=(2))
in_b = tf.placeholder(dtype=tf.float32, shape=(2))
def forward(x):
with tf.variable_scope("matmul", reuse=tf.AUTO_REUSE):
W = tf.get_variable("W", initializer=tf.ones(shape=(2,2)),
regularizer=tf.contrib.layers.l2_regularizer(0.04))
b = tf.get_variable("b", initializer=tf.zeros(shape=(2)))
return W * x + b
out_a = forward(in_a)
out_b = forward(in_b)
reg_loss = tf.losses.get_regularization_loss(scope="matmul")
with tf.Session() as sess:
sess.run(tf.global_variables_initializer())
outs = sess.run([out_a, out_b, reg_loss],
feed_dict={in_a: [1, 0], in_b: [0, 1]})
After conversion:
# Code in TF2.X (after conversion)
W = tf.Variable(tf.ones(shape=(2,2)), name="W")
b = tf.Variable(tf.zeros(shape=(2)), name="b")
@tf.function
def forward(x):
return W * x + b
out_a = forward([1,0])
out_b = forward([0,1])
regularizer = tf.keras.regularizers.l2(0.04)
reg_loss = regularizer(W)
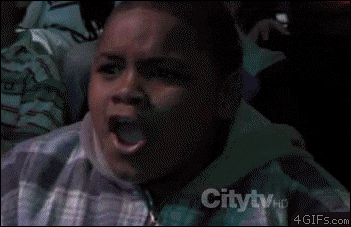
Happy Kerasing!